A version of this essay has been published by Open Magazine at https://openthemagazine.com/essays/the-new-knowledge-war/
Generative AI, as exemplified by chatGPT from Microsoft/OpenAI and Bard from Google, is probably the hottest new technology of 2023. Its ability has mesmerised consumers to provide answers to all sorts of questions, as well as to create readable text or poetry and images with universal appeal.
These generative AI products purport to model the human brain (‘neural networks') and are ‘trained’ on large amounts of text and images from the Internet. Large Language Models or ‘LLMs’ are the technical term for the tools underlying generative AI. They use probabilistic statistical models to predict words in a sequence or generate images based on user input. For most practical purposes, this works fine. However, in an earlier column in Open Magazine, “Artificial Intelligence is like Allopathy”, we pointed out that in both cases, statistical correlation is being treated by users as though it were causation. In other words, just because two things happened together, you can’t assume one caused the other. This flaw can lead to completely wrong or misleading results in some cases: the so-called ‘AI hallucination’.
To test our hypothesis, we asked chatGPT to summarise that column. It substantially covered most points, but surprisingly, though, it completely ignored the term ‘Ayurveda', although we had used it several times in the text to highlight ‘theory of disease’. This is thought-provoking, because it implies that in the vast corpus of data that chatGPT trained on, there is nothing about Ayurveda.
The erasure of Indic knowledge
Epistemology is the study of knowledge itself: how we acquire it, and the relationship between knowledge and truth. There is a persistent concern that Indic knowledge systems are severely under-represented or mis-represented in epistemology in the Anglosphere. Indian intellectual property is ‘digested’, to use Rajiv Malhotra’s evocative term.
For that matter, India does not receive credit for innovations such as Indian numerals (misnamed Arabic numerals), vaccination (attributed to the British, though there is evidence of prior knowledge among Bengali vaidyas), or the infinite series for mathematical functions such as pi or sine (ascribed to Europeans, though Madhava of Sangamagrama discovered them centuries earlier).
The West (notably, the US) casually captures and repackages it even today. Meditation is rebranded as ‘mindfulness’, and the Huberman Lab at Stanford calls Pranayama ‘cyclic sighing’. A few years ago, the attempts of the US to patent basmati rice and turmeric were foiled by the provision of ‘prior art’, such as the Hortus Malabaricus, written in 1676 about the medicinal plants of the Western Ghats.
Judging by current trends, Wikipedia, and presumably Google, LinkedIn, and other text repositories, are not only bereft of Indian knowledge, but also full of anti-Indian and specifically anti-Hindu disinformation. Any generative AI relying on this ‘poisoned’ 'knowledge base' will, predictably, produce grossly inaccurate output.
This has potentially severe consequences: considering that Sanskrit, Hindi, Tamil, Bengali (and non-Latin scripts) etc. are underrepresented on the Internet, generative AI models will not learn or generate text from these languages. For all intents and purposes, Indic knowledge will disappear from the discourse. These issues will exacerbate the bias against non-English speakers, who will not think about their identity or culture, reducing diversity and killing innovation.
More general problems with epistemology: bias, data poisoning and AI hallucinations
Generative AI models are trained on massive datasets of text and code. This means they are susceptible to inherent biases. A case in point: if a dataset is biased against non-white females, then the generative AI model will be more likely to generate text that is also biased against non-white women. Additionally, malicious actors can poison generative AI models by injecting false or misleading data into the training dataset.
For example, a coordinated effort to introduce anti-India biases into Wikipedia articles (in fact this is the case today) will produce output that is notably biased. An example of this is a query about Indian democracy to Google Bard: it produced a result that suggested this is a Potemkin construct (i.e., one that is merely a facade); Hindu nationalism and tight control of the media “which has become increasingly partisan and subservient to the government” were highlighted as concerns. This is straight from ‘toolkits', which have poisoned the dataset and are helped, in part, by US hegemonic economic dominance.
More subtly, generative AI models are biased towards Western norms and values (or have a US-centric point of view). For example, the Body Mass Index (BMI), a measure of body fat, has been used in Western countries to determine obesity, but is a poor measure for the Indian population, as we tend to have a higher percentage of body fat than our Western counterparts.
An illustration of AI hallucination came to the fore from an India Today story entitled "Lawyer faces problems after using ChatGPT for research. AI tool comes up with fake cases that never existed." It reported how a lawyer who used ChatGPT-generated precedents had his case dismissed because the court found the references were fabricated by AI. Similar risks in the medical field for patient treatment will be exacerbated if algorithms are trained on non-curated datasets.
While these technologies promise access to communication, language itself becomes a barrier. For instance, due to the dominant prevalence of English literature, a multilingual model might link the word dove with peace, but the Basque word for dove (‘uso’) is used as a slur. Many researchers have encountered the limitations of these LLMs, for other languages like Spanish or Japanese. ChatGPT struggles to mix languages fluently in the same utterance, such as English and Tamil, despite claims of 'superhuman' performance.
The death of Intellectual Property Rights
Intellectual property rights are a common concern. Already, generative AIs can produce exact copies (tone and tenor) of creative works by certain authors (for example, J K Rowling's Harry Potter series). This is also true of works of art. Two things are happening in the background: any copyright inherent in these works has been lost, and creators will cease to create original works for lack of incentives (at least according to current intellectual property theory).
A recent Japanese decision to ignore copyrights in datasets used for AI training (from the blog technomancers.ai, “Japan Goes All In: Copyright Doesn't Apply to AI Training”) is surprisingly bold for that nation, which moves cautiously by consensus. The new Japanese law allows AI to use any data “regardless of whether it is for non-profit or commercial purposes, whether it is an act other than reproduction, or whether it is content obtained from illegal sites or otherwise.” Other governments will probably follow suit. This is a land-grab or a gold rush: India cannot afford to sit on the sidelines.
India has dithered on a strict Data Protection Bill, which would mandate Indian data to be held locally; indirectly, it would stem the cavalier capture and use of Indian copyright. The Implications are chilling; in the absence of economic incentives, nobody will bother to create new works of fiction, poetry, non-fiction, music, film, or art. New fiction and art produced by generative AI will be Big Brother-like. All that we would be left with as a civilisation will be increasingly perfect copies of extant works: Perfect but soulless. The end of creativity may mean the end of human civilisation.
With AIs doing ‘creation’, will people even bother? Maybe individual acts of creation, but then they still need the distribution channels so that they reach the public. In the past in India, kings or temples supported creative geniuses while they laboured over their manuscripts, and perhaps this will be the solution: State sponsorship for creators.
Indian Large Language Models: too few yet, while others are moving ahead
Diverse datasets will reduce bias and ensure equitable Indic representation to address the concerns about generative AI. Another way is to use more rigorous training methods to reduce the risk of data poisoning and AI hallucinations.
Progressive policy formulations, without hampering technological developments, are needed for safe and responsible use to govern the use of LLM's across disciplines, while addressing issues of copyright infringement and epistemological biases. Of course, there is the question of creating ‘guardrails': some experts call for a moratorium, or strict controls, on the growth of generative AI systems.
We must be alive to its geopolitical connotations, as well. The Chinese approach to comprehensive data-collection is what cardiologists refer to as a ‘coronary steal phenomenon’: one segment of an already well-perfused heart ‘steals’ from another segment to its detriment. The Chinese, for lack of better word, plunder (and leech) data while actively denying market access to foreign companies.
Google attempted to stay on in China with Project Dragonfly, while Amazon, Meta, Twitter were forced to exit the market. Meanwhile, ByteDance, owner of TikTok, is trying to obscure its CCP ties by moving to a 'neutral jurisdiction' in Singapore, while siphoning off huge amounts of user data from Europe and the US (and wherever else it operates) for behavioural targeting and capturing personal level data, including from children and young adults. The societal implications of the mental health 'epidemic' (depression, low self-esteem, and suicide) remain profound and seem like a reversal of the Opium Wars the West had unleashed on China.
India can avoid Chinese exclusivism by keeping open access to data flows while insisting on data localisation. The Chinese have upped the ante. Reuters reported that “Chinese organisations have launched 79 AI large language models since 2020”, citing a report from their Ministry of Science and Technology. Many universities, especially in Southeast Asia, are creating new data sets to address the spoken dialects.
West Asia, possibly realizing the limitations of “peak-oil”, have thrown their hat in the ring. The United Arab Emirates (UAE) claims to have created the world’s “first capable, commercially viable open-source general-purpose LLM, which beats all Big Tech LLMs”. According to the UAE's Technology Innovation Institute, the Falcon 40B is not only royalty free, but also outperforms “Meta's LLaMA and Stability AI's StableLM”.
This suggests that different countries recognise the importance of investing resources to create software platforms and ecosystems for technological dominance. This is a matter of national security and industrial policy.
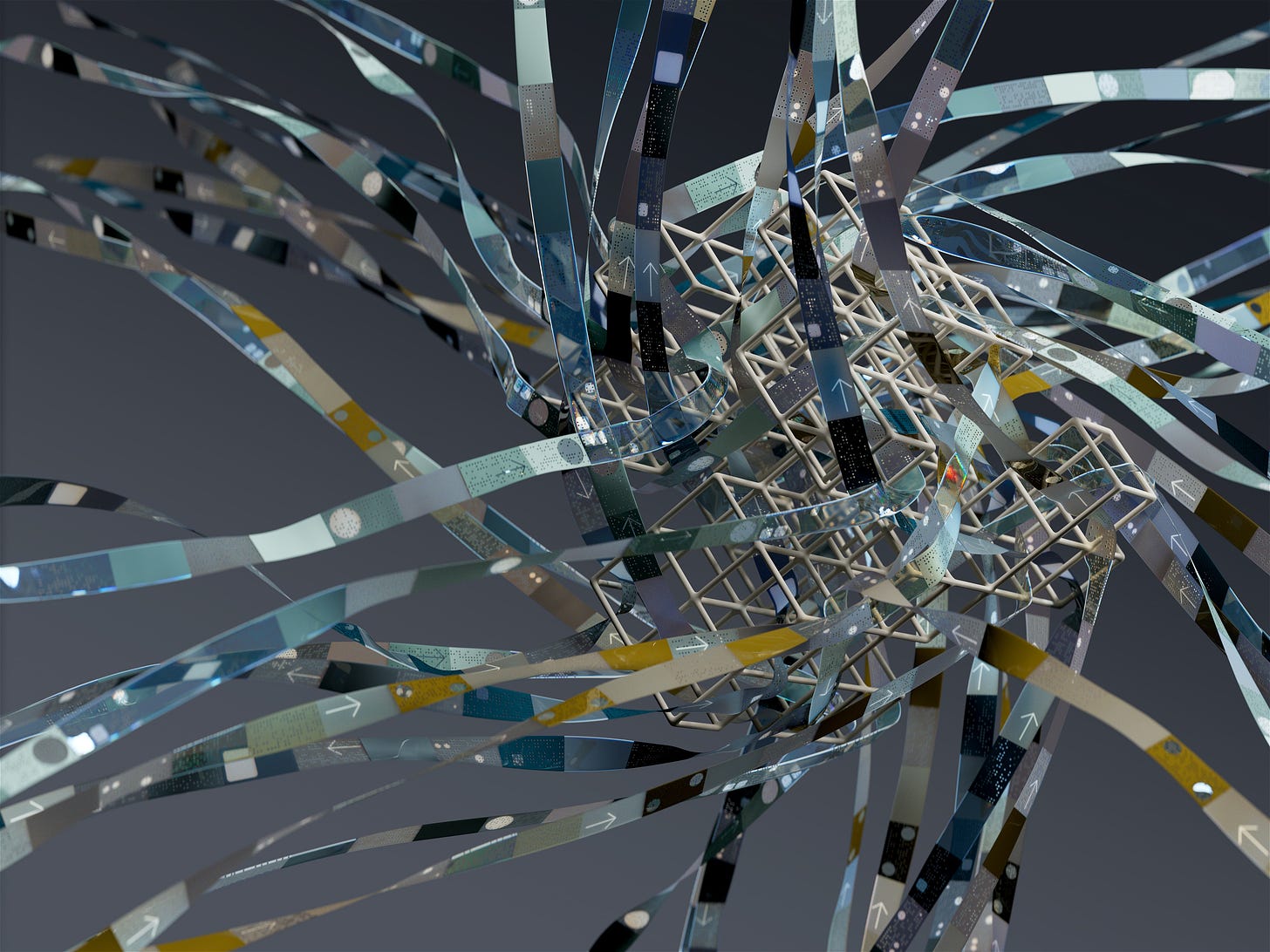
“We have no moat” changes everything: welcome to tiny LLMs
Chiranjivi from IIT Bombay, IndiaBERT from IIT Delhi and Tarang from IIT Madras are a few LLMs from India. India needs to get its act together to bring out many more LLMs: these can focus on, and be trained on, specialised datasets representing specific domains, for instance, that can avoid data poisoning. The Ministries concerned should provide support, guidance, and funding.
The obstacle has been the immense hardware and training requirements: GPT-3, the earlier generation LLM, required 16,384 Nvidia chips at a cost of over $100 million. Furthermore, it took almost a year to train the model with 500 billion words, at a cost of hundreds of millions of dollars. There was a natural assumption: the larger the data set, the better the result with ‘emergent’ intelligence. This sheer scale of investments was considered beyond Indian purview.
A remarkable breakthrough was revealed in a leaked internal Google memo, timed with Bard's release, titled "We have no moat, and neither does OpenAI," a veritable bombshell. It spoke about Meta’s open sourcing its algorithmic platform, LLaMA, and implications for generative AI research. Although there is no expert consensus, the evidence suggests smaller datasets can produce results almost as good as the large datasets.
This caused a flutter among the cognoscenti. Despite Meta releasing its crown jewels for a wider audience (developers), there was an uptick in its stock value, despite failures in its multiple pivots beyond social media.
To understand this better, Geoffrey Hinton, the ‘godfather’ of deep learning, explains in detail: All large language model (LLM) copies can learn separately, but share their knowledge instantly. That’s how chatbots know more than an average person. The performance trajectory of different LLM’s has skyrocketed; for example, consider this:
Using LLaMa as a base, researchers were able to quickly (in weeks) and cheaply (a few $100) produce Alpaca and Vicuna that, despite having fewer parameters, compete well with Google’s and openAI's models. The graph shows that the answers from their chatbots are comparable in quality (per GPT-4). A fine-tuning technique called LoRA (Low Rank Adoption) is the secret behind this advance.
This abruptly levels the playing field. Open-source models can be quickly scaled and run on even laptops and phones! Hardware is no longer a constraint. Let a thousand Indian LLMs bloom!
The way forward
Given the astonishing amounts being invested by venture capitalists and governments in generative AI, there will be an explosion in startup activity. There are already a few in India, such as Gan, Kroopai, Peppertype.ai, Rephrase.ai, TrueFoundry, and Cube. Still, TechCrunch quoted Sanford Bernstein analysts who painted a gloomy picture: “While there are over 1500 AI-based startups in India with over $4 billion of funding, India is still losing the AI innovation battle”.
Without exaggeration, it can be argued that this is an existential threat for India, and needs to be addressed on a war-footing. The AIforBharat initiative at IIT Madras is a start, but much more is needed. A sharply focused set of policies and regulations needs to be implemented by the government immediately that will both prevent the plunder of our intellectual property and data, and also encourage the creation of large numbers of models that make good use of Indian ingenuity and Indic knowledge.
2245 words, 4 June 2023
Ep. 104: If India does not encourage and regulate Artificial Intelligence innovation, it could be game over